
Diagnosing Alzheimer’s Disease with Disruptive Advances: Artificial Intelligence Knows No Limits
The early detection of AD based on AI would allow easier management for care providers and the patient’s family, thus decreasing financial expenses and overall healthcare costs to treat this pathology.
“Clearly, the thing that’s transforming is not the technology - it’s the technology that is transforming you.” (Jeanne W. Ross)
In a previous article, I talked about aging, discussing how, in certain cases, this biological process may deviate from its normal course, manifesting the occurrence of pathologies. In particular, I focused on describing Alzheimer’s disease (AD), being one of the most common diseases that emerge with aging and the sixth leading cause of death in the US. Considering AD a real social concern, besides the lack of effective and established treatments to arrest the progression of the disease, I reported a few studies in which advanced technologies have been implemented to identify digital biomarkers. Hence, advanced methods were demonstrated to detect early disease onset, thus creating an opportunity to transform disease insights into actions. Hope for the future of health across the aging population.
In a series of two articles, we will continue this journey into the understanding of AD, and where innovative technologies such as artificial intelligence (AI), gene therapies, virtual reality, (VR) nasal vaccines have been recently implemented to diagnose and treat AD. In addition, the global threat of underpopulation and increased shift toward the aging population will also be discussed. In particular, I will examine several scientific findings in which vaccines developed to kill senescent cells, and outstanding DNA gene therapies were recently proposed to reverse aging and treat age-related diseases.
Aging, memory, and learning impairments
Based on the World Health Organization (WHO), it is estimated that 50 million people are diagnosed with dementia worldwide, with an increasing rate of 10 million each year. Among dementia cases, AD is the most common type, accounting for 60-70% of dementia cases. Indeed, AD is one of the main causes of death in the US, and 122,0019 Americans died in 2018 due to AD. Furthermore, it can be predicted that by 2050 individuals older than 60 are going to double, thus increasing the possible number of AD cases, with a consequent negative effect on the entire healthcare system [1].
So far, a specific treatment to stop or delay the pathology has not been found yet. Hence, the research aims to identify those biomarkers predictors of the disease's progression that can detect the pathology even 25 years before any symptoms are visible. However, identifying early signs with cost-effective and non-invasive methods is a great challenge for any healthcare provider. The diagnosis of AD has been traditionally made based on a series of lab tests, medical history, and patient information. However, when cognitive symptoms occur (e.g., memory complaints, attention deficits, poor reasoning, impaired communication, confusion) is already late, and the structural brain damage cannot be reversed. Therefore, to detect early AD signs and disease progression, among innovative methods, AI technology may represent a possible solution while supporting physicians in making accurate predictions that were not even possible years ago [1].
In this regard, AI systems are trained over large datasets made of millions of electronic records, genetic information, health care claims, socio-demographic information, lifestyle, personal, and environmental variables to establish relationships between data and factors that apparently appear unrelated and not significant. However, to make accurate predictions, the need for data integrity is a requisite for any AI algorithms. Algorithms are then trained with a variety of clinical, genetic, as well as, imaging data based on positron emission tomography (PET) and magnetic resonance imaging (MRI) scans to categorize between groups of normal individuals and those who present clinical AD features, and thus diagnosed with AD or other cognitive complaints. This procedure gives physicians the benefit of spotting AD six years earlier than traditional methods, so treating the pathology before the spread of progressive volume loss that otherwise would become irreversible. In addition, the early detection of AD based on AI would allow easier management for care providers and the patient’s family, thus decreasing financial expenses and overall healthcare costs to treat this pathology [1].
AI can spot and predict AD early over time
Brain activity is the result of billions of neurons that constantly interact while translating this activity to generate thoughts, behaviors, and cognitive functioning. Among neuroimaging techniques, the most common are functional magnetic resonance imaging (fMRI) which measures the activity of the brain based on the blood flow change (BOLD signal), and calcium imaging (CaI) which detect the activity of neurons over time to differentiate the functioning of neuronal circuits. Moreover, the implementation of algorithms on imaging data, such as AI systems, can be useful to make accurate predictions based on imaging recognition. Nevertheless, to train and test AI algorithms, a considerable amount of different datasets is needed to provide reliable insights about brain mechanisms, as well as, the development of these models can also be combined with brain-computer interface (BCI) technologies. However, while mapping the brain, measuring and modeling this activity is a complex procedure. Indeed, neural activity is not linear, and human behavior is unpredictable but not random. In addition, when training neural networks (e.g., deep RNNs) to predict patterns accurately, a great amount of data is needed, although the availability of big imaging datasets is limited [2].
Hence, a hybrid approach to building an AI model called “VanDEEPol” was recently proposed by IBM. This model combines nonlinear van der Pol (VDP) oscillations with an RNN to predict neural activities measured with imaging data. VDP oscillations can fit data of different species and generalize on untrained data while identifying how brain areas interact, thus gaining insight into functional connectivity, as well as, providing unlimited simulated data to improve real imaging information used to train an RNN [2]. Fitting multivariate brain imaging parameters and predicting time-series data with precision and great accuracy, the potential of the VDP model is outstanding, also used to improve the performance of deep RNNs. The importance of VDP to predict brain activity based on imaging measures can help physicians in diagnosing a variety of medical disorders while predicting the progression of the condition [2], but also BCIs might be implemented to restore brain functioning that is impaired due to aging or other disabilities.
Supporting physicians to improve therapies with AI
The importance of implementing AI to improve therapies while supporting physicians has been widely recognized. A recent study, published in the Journal of Pharmacokinetics and Pharmacodynamics, did show that AI can effectively be implemented to detect and predict diseases, particularly those conditions that arise with aging. Thus, scientists from the State University of New York, University of Buffalo are aimed to develop models in which biomarkers of metabolic and cardiovascular disease are going to be used to investigate biological processes in the human body to predict health conditions based on specific indicators (e.g., glucose, blood pressure, cholesterol level, and body max index). This system based on AI will be able to measure the risk for developing digestive and respiratory diseases, but also to help physicians the monitoring of long-term chronic drug therapy used to treat diabetes, high cholesterol, and blood pressure diseases.
Due to the capability of AI to analyze and manage big data to further investigate, diagnose, treat, and prevent diseases, it may represent hope when exploring neurodegenerative diseases such as AD. Indeed, effective treatments in stopping or even delaying the pathology have failed in their mission, forcing research to develop innovative methods. Thus, Gramberger et al. (2017) [3] applied a new unbiased AI algorithm called “multilayer clustering” to analyze several data points based on the “Alzheimer’s Disease Neuroimaging Initiative”, which was a US study that included cognitive tests, brain imaging data, and spinal fluid biomarkers. The sample was based on 562 individuals diagnosed with mild cognitive impairment (MCI), followed for 5 years. Results showed that AI was able to distinguish two groups of individuals: those at high risk for developing AD due to their rapid cognitive decline, and those who did not show hallmark symptoms of rapid cognitive decline. However, also a third group has been classified who remained unclustered. These findings did also show that those who demonstrated a rapid decline had a greater level of brain atrophy, progressing from MCI to dementia at a rate that was five-time faster than those who showed a minor decline and with a slower disease progression. Such results implicate that, while grouping individuals with similar features (e.g., brain changes, cognitive impairments), AI systems, particularly unbiased algorithms, play a relevant role in supporting physicians to detect those patients that are at risk for developing AD, even years before the pathology can be clinically diagnosed [3].
AD is heterogeneous but AI can categorize its subtypes
Furthermore, AD is a complex disease characterized by the spread of tau pathology across the cerebral cortex. However, recent studies demonstrated that this brain pattern is heterogeneous. From its clinical manifestation to its structural pathology, a considerable variability among patients is mostly observed, and where also atypical forms were presented. Thus, AD and its subtypes become hard to properly diagnose, leading to a lack of consensus across the scientific community. What is idiopathic AD? Is there a “typical” AD type? Research led by Oskar Hansson at Lund University in Sweden was able to demonstrate the powerfulness of AI to distinguish among four types of AD.
In the study, the largest dataset of tau-PET data was analyzed. The machine learning (ML) method applied over thousands of imaging scans from 1,612 individuals, was able to differentiate four AD types based on four evident spatiotemporal trajectories of tau pathology. Indeed, each subtype showed a different progression of neurofibrillary tangle pathology over different regions, defying distinct clinical profiles and rates of disease progression [4]. These subtypes were demonstrated to be stable during a follow-up study and even replicated in a separate sample in which a different radiotracer was used. These results indicated the variability of tau pathology among patients is systematic and in which, the pathology originates and diffuses across different and specific corticolimbic networks for each subtype. Therefore, a novel examination of the “typical AD” definition, as well as, the stage of tau progression should be revised [4]. “This is the kind of paper that can change a field,” “we know that not all AD patients are equal and that they don’t have exactly the same set of symptoms or trajectories, but the power of their model is that they can explain both the symptoms and the progression of the disease,” said Bernard Hanseeuw, Massachusetts General Hospital (Alzforum).
Investing in AI technologies to promote healthy aging
Looking at future trends, an outstanding project financially supported by Bill Gates is aimed to develop a predictive device in diagnosing earlier stages of AD, and possibly approved by the US Food and Drug Administration (FDA) [5]. In collaboration with Eisai and the Bioinformatics and Human Electrophysiology Laboratory (BiHeLab) at Ionian University), Altoida initiated a five-year AD multicentre study based on an AI software device to evaluate thousands of patients at different stages of the disease. Indeed, BiHeLab is a research center dedicated to the implementation of mathematical modeling and ML specifically to investigate neurodegenerative disorders with analytical methods. Thus, Altoida would take the advantage to adopt an AI-based device to measure the outcome of digital tests, presented on tablets or mobile phones, in patients to spot AD at its earlier stages [5]. In addition, these digital tests are combined with augmented reality (AR) to simulate simple daily living tasks. To enhance the validity of this newly developed device in diagnosing early cognitive decline, digital biomarkers and clinical data (e.g., imaging data, genomics) will also be evaluated. Lastly, Altoida hopes that this study will represent a breakthrough in the study of AD while enhancing and better clarifying the understanding of AD pathology [5].
Promoting healthy aging with AI-based technologies becomes a necessity to foster in the coming years; an investment that the National Institute of Aging has already allocated to fund Johns Hopkins in pursuing this research. Hence, research and the experimental environment from the Johns Hopkins University schools of medicine and nursing, the Whiting School of Engineering, ad the Carey Business School, will dedicate the received grant of over $20M to build an AI system and technology collaboratory (AITC), aimed at ameliorating the lifestyle and quality of life of the aging population. Indeed, “the excitement is that our work can help physicians use the technology as markers for measuring the evolution of age-related diseases, like dementia and Alzheimer’s, and predicting falls using patterns and behaviors of older adults,” said Rama Chellappa, professor of electrical and computer engineering. A newly social, and scientific initiative to enhance awareness toward the potential of AI advances among citizens while promoting public health through technologies.
Solving underpopulation and age-related diseases with gene therapy, vaccines, VR, and much more
Having seen how AI algorithms can become a game-changer in detecting and diagnosing AD, raising hope in the possibility of blocking disease progression, in the next article I will talk about other innovative findings in which gene therapies, vaccines, and VR technologies have been implemented to treat AD. Moreover, the global concern of underpopulation due to reduced fertility rates, with a consequent demographic shift toward aging will also be discussed. Scientific evidence regarding gene therapies to reverse our biological clock, as well as, to prevent age-related diseases will be reviewed concerning healthy longevity, and abundance for humanity.
“Data is the new science. Big Data holds the answers.” (Pat Gelsinger)
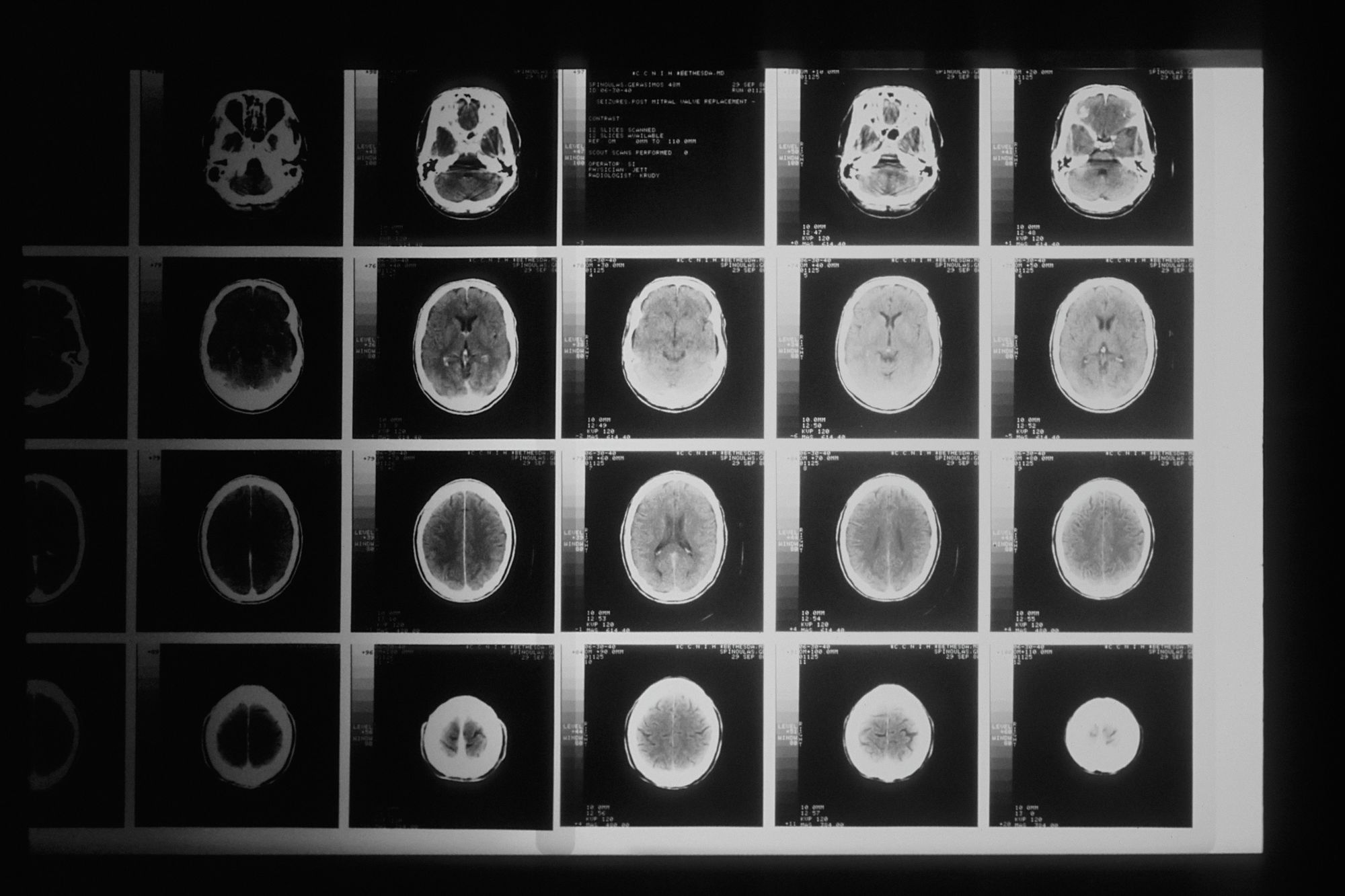
References:
- Dongmin, K. Imaging Informatics: How AI Is Tackling Alzheimer’s Disease. Radiology Today, Vol. 20 No. 11 P. 6. Available at: https://www.radiologytoday.net/archive/rt1119p6.shtml [Accesssed on December 13, 2021]
- Gandharv, K. (2021). IBM’s AI Model To Predict Brain Activity, Helps Detect Medical Disorders. Available at: https://analyticsindiamag.com/ibms-ai-model-to-predict-brain-activity-helps-detect-medical-disorders/ [Accessed on December 12, 2021]
- Gamberger, D., Lavrač, N., Srivatsa, S. et al. Identification of clusters of rapid and slow decliners among subjects at risk for Alzheimer’s disease. Sci Rep 7, 6763 (2017). https://doi.org/10.1038/s41598-017-06624-y
- Vogel JW, Young AL, Oxtoby NP, Smith R, Ossenkoppele R, Strandberg OT, La Joie R, Aksman LM, Grothe MJ, Iturria-Medina Y; Alzheimer’s Disease Neuroimaging Initiative, Pontecorvo MJ, Devous MD, Rabinovici GD, Alexander DC, Lyoo CH, Evans AC, Hansson O. Four distinct trajectories of tau deposition identified in Alzheimer's disease. Nat Med. 2021 May;27(5):871-881. doi: 10.1038/s41591-021-01309-6
- NS Medical Devices (2021). Altoida begins multicentre Alzheimer’s study with AI-based device. Available at: https://www.nsmedicaldevices.com/news/altoida-alzheimers-study-ai-device/ [Accessed on December 13, 2021]
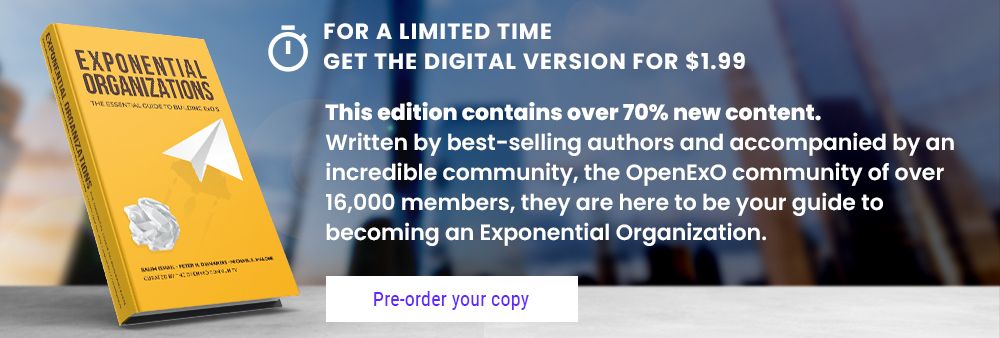
ExO Insight Newsletter
Join the newsletter to receive the latest updates in your inbox.